From Siri to self-driving cars, AI is all around us. But not all AI is created equal. In 2024, understanding the different types of AI is crucial for businesses and individuals alike. Whether you're looking to improve efficiency, automate processes, or gain a competitive edge, knowing the right type of AI can make all the difference.
So, what are the 7 types of AI you need to know? From narrow AI that excels at specific tasks to the elusive general AI that can think like a human, we’ll break down each type and how they're being used today. Plus, we'll explore the key differences between rule-based and learning-based AI, supervised and unsupervised learning, and more. Get ready to dive into the world of AI and discover which types can help you achieve your goals in 2024 and beyond.
Types of AI: Narrow AI vs. General AI

Narrow AI is the most common type of AI today, focused on specific tasks, while General AI, also known as AGI, can perform any intellectual task like a human. Understanding the difference between narrow and general AI is crucial for businesses.
Narrow AI: Focused on Specific Tasks
Narrow AI, also known as weak AI or artificial narrow intelligence (ANI), is designed to perform a single specific task exceptionally well. It operates within a predefined set of parameters and cannot generalize its knowledge to other areas. Examples of narrow AI include:
- Voice Assistants: Siri and Alexa, which can understand and respond to voice commands.
- Self-Driving Cars: Vehicles that can navigate roads and avoid obstacles.
- Recommendation Systems: Used by Netflix and Amazon to suggest content.
Narrow AI is the most common type of AI in use today. It has proven to be highly effective in automating repetitive tasks, analyzing large datasets, and making predictions based on historical data. However, narrow AI cannot think or reason like a human, and it lacks the ability to learn from its experiences and apply that knowledge to new situations.
Advantages of Narrow AI:
- Increased Efficiency: Automating tasks that would otherwise require human intervention helps businesses save time and resources.
- Improved Accuracy: Narrow AI can process vast amounts of data quickly and accurately, reducing the risk of human error.
- Cost Savings: Implementing narrow AI solutions can help businesses reduce labor costs and increase productivity.
General AI: Able to Perform Any Intellectual Task
General AI, also known as strong AI or artificial general intelligence (AGI), refers to a hypothetical machine that can think, learn, and apply knowledge just like a human. AGI would be able to perform any intellectual task that a human can, from writing poetry to solving complex mathematical problems.
Unlike narrow AI, which is limited to specific tasks, AGI would possess the ability to reason, plan, and make decisions based on its accumulated knowledge and experiences. It would be able to learn from its mistakes and adapt to new situations, much like a human brain.
However, AGI remains a theoretical concept and has not yet been achieved. Researchers and experts disagree on when, or if, AGI will become a reality. Some believe that AGI is decades away, while others argue that it may never be possible to create a machine that can truly think like a human.
Potential Impact of AGI:
- Automation of Complex Tasks: AGI could automate jobs that require human-level intelligence, such as doctors, lawyers, and scientists.
- Accelerated Scientific Discovery: AGI could help solve complex problems and accelerate research in fields like medicine, physics, and engineering.
- Ethical Concerns: The development of AGI raises ethical questions about the role of machines in society and the potential risks of creating a superintelligent entity.
As businesses continue to adopt AI technologies, understanding the difference between narrow AI and general AI is crucial for making informed decisions about how to leverage these tools for growth and innovation. While narrow AI is already transforming industries, the development of AGI remains an open question with significant implications for the future of work and society as a whole.
Just a note to understand the differences, self-driving cars, while sophisticated, are considered Narrow AI because they are specifically designed to perform the task of driving. They use sensors, cameras, machine learning algorithms, and data processing to navigate roads, avoid obstacles, and make driving decisions in real-time. However, their intelligence is limited to this particular domain—they cannot generalize their knowledge to perform tasks outside of driving. For example, a self-driving car cannot use its "knowledge" to perform tasks like playing chess, writing a poem, or having a conversation.
In contrast, General AI (or AGI) would possess the ability to learn, understand, and apply knowledge across a wide range of tasks, similar to human intelligence. General AI could theoretically drive a car, play chess, write poetry, and more, all with the same underlying intelligence. However, such AI has not yet been developed and remains a theoretical concept.
So, in summary, self-driving cars are a prime example of Narrow AI due to their specialized functionality in the domain of autonomous driving.
Types of AI: Rule-Based vs. Learning-Based AI

Rule-based AI follows predefined rules, while learning-based AI learns from data. Rule-based AI is less flexible but easier to understand and control, whereas learning-based AI can adapt and improve over time but requires large amounts of data.
Rule-Based AI: Follows Predefined Rules
Rule-based AI systems rely on a set of human-coded rules and logic to make decisions and perform tasks. These rules are explicitly programmed by experts in the domain, and the AI system follows these rules precisely to arrive at conclusions or take actions.
Examples of rule-based AI include expert systems, which are designed to mimic the decision-making process of a human expert in a specific field, such as medical diagnosis or financial planning. These systems use a knowledge base of if-then rules to analyze information and provide recommendations. Chatbots with predefined responses are another example of rule-based AI, where the system matches user input to predefined patterns and provides corresponding responses.
The main advantage of rule-based AI is that it is relatively easy to understand and control, as the rules are explicitly defined. However, this also means that rule-based AI systems are less flexible and cannot easily adapt to new situations or learn from experience.
Limitations of Rule-Based AI:
- Requires Extensive Human Expertise: Creating and maintaining rules requires significant expertise.
- Limited Ability to Handle Complex or Ambiguous Situations: Rule-based AI struggles with scenarios not covered by its predefined rules.
- Cannot Learn or Improve Performance Over Time: Rule-based AI systems cannot adapt or improve based on new data.
Learning-Based AI: Learns from Data
In contrast, learning-based AI systems use machine learning algorithms to learn patterns and relationships from data. Instead of being explicitly programmed with rules, these systems are trained on large datasets and learn to make predictions or decisions based on the patterns they discover.
The two main types of learning-based AI are supervised learning and unsupervised learning. Examples of learning-based AI include neural networks and deep learning models, which are used in a wide range of applications such as image recognition, natural language processing, and predictive analytics.
Benefits of Learning-Based AI:
- Can Discover Complex Patterns and Relationships in Data: Learning-based AI can identify patterns that are not easily captured by predefined rules.
- Adaptable to New Situations: These systems can improve performance over time as they are exposed to more data.
- Suitable for Tasks Where Explicit Rules Are Difficult to Define: Learning-based AI is ideal for complex or dynamic environments.
Types of AI: Supervised vs. Unsupervised Learning
Supervised and unsupervised learning are two fundamental approaches in AI, with supervised learning using labeled data to train AI models for specific tasks, and unsupervised learning allowing AI to discover patterns in unlabeled data.
Supervised Learning: Learning from Labeled Data
In supervised learning, the AI system is trained using labeled data, where the input data is accompanied by the correct output or target. The goal is for the AI to learn a function that maps the input data to the correct output labels. This allows the AI to make predictions or decisions based on new, unseen data.
The training process in supervised learning involves providing the AI with a large dataset of input-output pairs. For example, in an image classification task, the input data would be a set of images, and the output labels would indicate the object or category each image belongs to, such as "cat," "dog," or "car." The AI learns to recognize patterns and features in the input data that are associated with each output label.
Common Examples of Supervised Learning:
- Image Classification: Assigning labels to images based on their content.
- Sentiment Analysis: Determining the sentiment (positive, negative, or neutral) of a piece of text.
- Fraud Detection: Identifying fraudulent transactions based on historical data.
Training and Testing in Supervised Learning: The supervised learning process typically involves splitting the labeled dataset into two subsets: a training set and a testing set. The AI model is trained on the training set, where it learns to map inputs to the correct outputs. After training, the model's performance is evaluated on the testing set, which contains data the model has not seen before. This helps assess how well the model generalizes to new data.
Unsupervised Learning: Discovering Patterns in Unlabeled Data
In contrast, unsupervised learning deals with unlabeled data, where the AI system is not provided with any target outputs or labels. The goal of unsupervised learning is to discover hidden structures, patterns, or relationships within the input data.
Unsupervised learning algorithms aim to identify inherent groupings or clusters in the data based on similarities or differences between data points. The AI system learns to represent the data in a way that captures its underlying structure without being explicitly told what to look for.
Common Applications of Unsupervised Learning:
- Customer Segmentation: Grouping customers based on their purchasing behavior or demographics.
- Anomaly Detection: Identifying unusual or outlier data points that deviate from the norm.
- Dimensionality Reduction: Reducing the number of features in high-dimensional data while preserving its essential structure.
Clustering and Dimensionality Reduction Techniques: Two popular techniques in unsupervised learning are clustering and dimensionality reduction. Clustering algorithms, such as k-means or hierarchical clustering, group similar data points together based on their proximity or similarity in the feature space. This can help discover natural groupings or segments within the data.
Dimensionality reduction techniques, like Principal Component Analysis (PCA) or t-SNE, aim to reduce the number of features in high-dimensional data while retaining the most important information. This can help visualize and explore complex datasets, as well as improve the efficiency of subsequent learning tasks.
Combining Supervised and Unsupervised Learning
While supervised and unsupervised learning are distinct approaches, they can also be combined in various ways to solve complex problems. For example, unsupervised learning can be used as a preprocessing step to discover meaningful features or representations in the data, which can then be used as input for a supervised learning task. This approach is known as feature learning or representation learning.
Another way to combine these approaches is through semi-supervised learning, where a small amount of labeled data is used in conjunction with a large amount of unlabeled data. The labeled data helps guide the learning process, while the unlabeled data allows the AI to discover additional patterns and structures that may not be captured by the labeled examples alone.
As AI continues to evolve, understanding the differences and complementary nature of supervised and unsupervised learning will be crucial for developing effective and efficient AI systems across a wide range of applications.
Generative AI vs. Predictive AI: Expanding the Scope of AI
In addition to understanding the key types of AI, it's important to recognize the difference between Generative AI and Predictive AI, as these two approaches represent different ways that AI systems can interact with and interpret data.
Generative AI is a type of AI that creates new content based on the data it has been trained on. It can generate images, text, music, and more, mimicking the patterns found in the training data. For example, Generative AI models like GPT (Generative Pre-trained Transformer) can write essays, create poetry, or simulate human conversation by generating new text that resembles the input data. Generative AI is widely used in creative industries, content generation, and even in creating synthetic data for various applications.
Predictive AI, on the other hand, focuses on analyzing existing data to make predictions about future events or trends. This type of AI is commonly used in fields such as finance, healthcare, and marketing. For example, a predictive AI model might analyze historical sales data to forecast future sales, or it might use patient data to predict the likelihood of developing certain medical conditions. Predictive AI is essential for decision-making processes where forecasting future outcomes based on past data is critical.
Key Differences:
- Purpose: Generative AI is used to create new content, while Predictive AI is used to anticipate future events or trends.
- Output: Generative AI produces new data (e.g., images, text), whereas Predictive AI provides insights or forecasts based on existing data.
- Applications: Generative AI is often employed in creative fields, while Predictive AI is more commonly used in analytical and decision-making processes.
Other Notable Types of AI
Reactive machines, limited memory AI, and theory of mind AI are less common but still important AI types. These AI systems have unique capabilities and limitations that set them apart from other AI categories. Understanding these AI types helps provide a comprehensive view of the AI landscape in 2024.
Reactive Machines
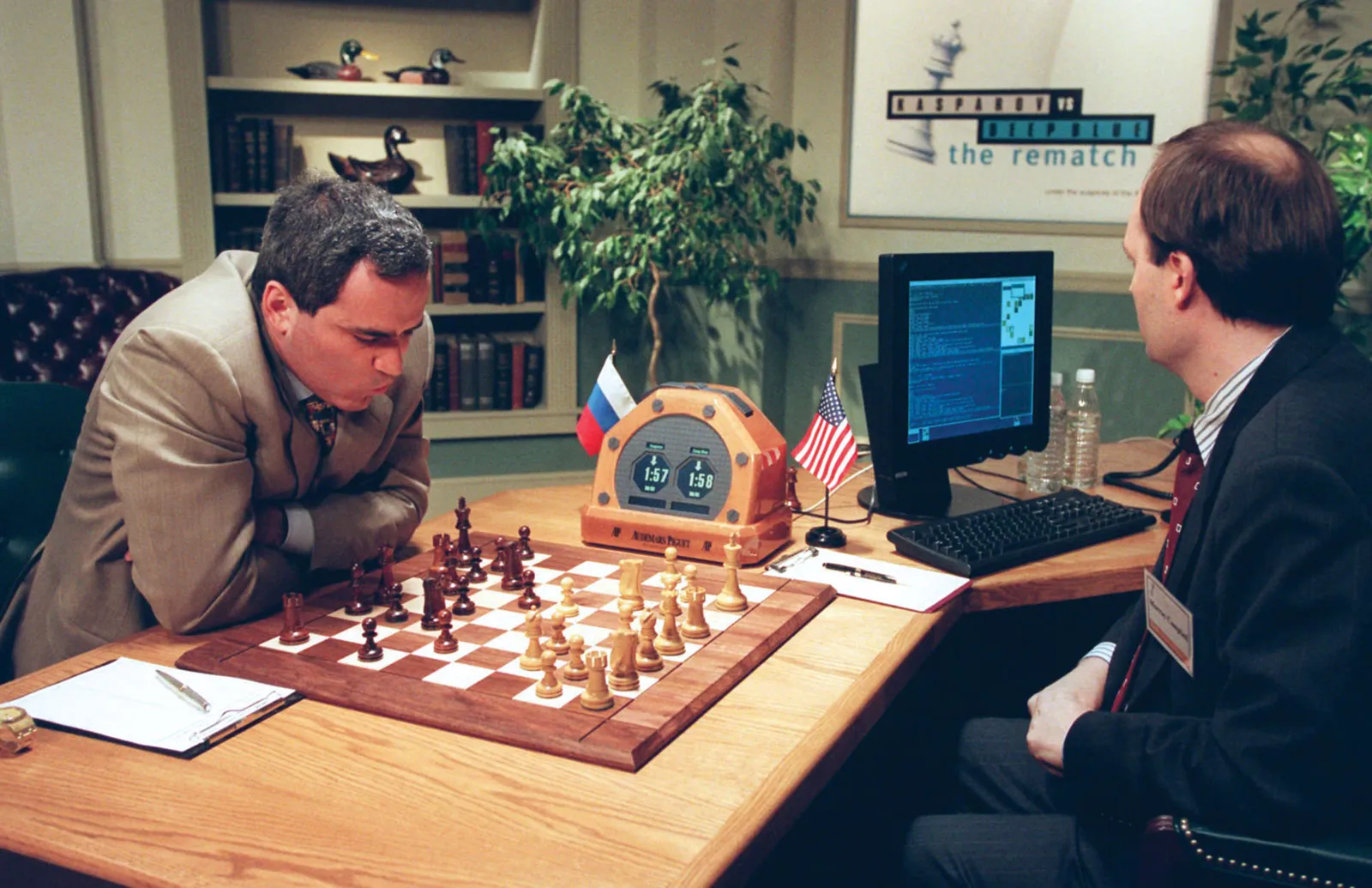
Reactive machines are a type of AI that can only respond to current inputs and have no memory of past events. These systems are designed to perform specific tasks based on the information they receive in the present moment. They do not have the ability to learn from experience or adapt their behavior based on previous interactions.
A well-known example of a reactive machine is IBM's Deep Blue chess-playing computer, which famously defeated world champion Garry Kasparov in 1997. Deep Blue relied on a vast database of chess moves and positions, along with powerful processing capabilities, to analyze the current state of the chessboard and determine the best move. However, it had no memory of previous games or the ability to learn from its experiences.
Reactive machines are still used today in various applications where rapid decision-making based on current inputs is required, such as in manufacturing process control systems or real-time fraud detection in financial transactions. Simple chatbots and recommendation systems also rely on reactive machine principles to provide immediate responses based on user input.
Limited Memory AI
Limited memory AI systems represent a step up from reactive machines in terms of complexity and capability. These systems can use past experiences to inform their current decisions and actions. They have a limited form of memory that allows them to retain information about previous events and use that knowledge to guide their behavior.
One of the most prominent examples of limited memory AI is found in self-driving cars. These vehicles use a combination of sensors, cameras, and machine learning algorithms to navigate roads safely. They can store data about past driving experiences, such as road conditions, traffic patterns, and obstacles encountered, and use that information to make better decisions in real-time.
Another example of limited memory AI is found in chatbots that can maintain context awareness during a conversation. These chatbots can remember previous user inputs and use that information to provide more relevant and personalized responses as the conversation progresses.
However, it's important to note that the memory in these systems is still limited in scope and duration. They cannot retain information indefinitely and may struggle with tasks that require a deep understanding of long-term dependencies or complex reasoning.
Theory of Mind AI
Theory of mind AI represents a more advanced and complex form of artificial intelligence that is still largely in the research phase. The concept of theory of mind refers to the ability to understand that other entities, whether human or artificial, have their own beliefs, intentions, and desires that may differ from one's own.
In the context of AI, a system with theory of mind would be able to infer the mental states of other agents and use that understanding to guide its own actions and decisions. This type of AI would require a deep understanding of social interactions, emotions, and the ability to model the thought processes of others.
While there have been some promising developments in this area, such as the creation of AI agents that can engage in simple forms of social reasoning or emotional understanding, true theory of mind AI has not yet been fully realized in practical applications. Researchers at MIT's Media Lab and DeepMind are actively exploring the development of theory of mind AI through projects like multi-agent systems and social reasoning experiments.
The Challenges of Developing Theory of Mind AI: Developing AI systems with theory of mind capabilities presents significant challenges for researchers and engineers. Some of the key hurdles include:
- Modeling Complex Social Interactions: Understanding the nuances of human communication.
- Representing and Reasoning About Beliefs and Emotions: Accurately modeling the mental states of other agents.
- Integration with Other Aspects of AI: Combining theory of mind with perception, learning, and decision-making.
- Ethical and Responsible Development: Ensuring ethical considerations in the development and deployment of theory of mind AI systems.
Despite these challenges, the potential benefits of theory of mind AI are significant. Such systems could revolutionize fields like education, mental health, and human-robot interaction by enabling more natural and empathetic interactions between AI and humans.
As we continue to explore the different types of AI and their capabilities, it's clear that the field of artificial intelligence is rapidly evolving. From reactive machines to limited memory AI and the ongoing research into theory of mind, each type of AI has its own unique strengths and limitations. Understanding these distinctions is crucial for developers, researchers, and users alike as we navigate the complex landscape of AI in 2024 and beyond.
Embracing the AI Revolution
As we've explored the seven key types of AI, it's clear that artificial intelligence is no longer a futuristic concept but a reality that is shaping our world in profound ways. From narrow AI applications that excel at specific tasks to the theoretical potential of general AI, the field of AI is diverse and constantly evolving.
By understanding the differences between rule-based and learning-based AI, as well as supervised and unsupervised learning, you're now equipped with the knowledge to navigate the AI landscape and make informed decisions about how to leverage these technologies in your business.
So, what's the next step in your AI journey? Start by identifying areas within your organization where AI can make a meaningful impact. Whether it's automating repetitive tasks, gaining insights from large datasets, or enhancing customer experiences, there are countless opportunities to harness the power of AI.
As you embark on this path, remember to stay curious, adaptable, and open to the possibilities that AI presents. The future belongs to those who can effectively integrate AI into their strategies and operations. Are you ready to embrace the AI revolution and unlock new frontiers of innovation and growth?